Translate this page into:
Bayesian instrument for spinal disability: A predictive model for aircrew flying fitness
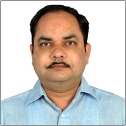
*Corresponding author: Punyashlok Biswal, Institute of Aerospace Medicine, Indian Air Force, Bengaluru, Karnataka, India. drpunyashlok@gmail.com
-
Received: ,
Accepted: ,
How to cite this article: Biswal P, Narayanappa D. Bayesian instrument for spinal disability: A predictive model for aircrew flying fitness. Indian J Aerosp Med 2024;68:55-60. doi: 10.25259/IJASM_23_2024
Abstract
Objectives
Spinal disabilities are a common problem in aircrew with or without obvious pathology in the spine. Department of Human Engineering, Institute of Aerospace Medicine (IAM) evaluates Indian air force (IAF) aircrew with spinal disabilities before they can fly again. This study aimed to develop a Bayesian Instrument for Spinal disability (BIS) based on clinical, radiological, and functional evaluations of aircrew at IAM. This research aims to develop a composite model of spinal disability assessment applicable to aircrew of different streams and to assess key factors that determine flying fitness. The study also aims to develop a computerized version of the scale that can be used in the field before referral to IAM.
Material and Methods
120 aircrews reporting spinal-related disabilities to the Department of Human Engineering, IAM participated in the study. 14 predictors that determine the return to flying in an aircrew were incorporated in a Bayesian Belief Network using an Augmented Naïve Bayes algorithm to develop the BIS. The predictive characteristics of the final model were independently tested on an incomplete data set of 29 aircrews.
Results
Validation of the spinal disability scale was carried out using the Leave One Out method. The accuracy was found to be 0.97, sensitivity was 0.97, and specificity was 0.96. The spinal disability scale was also tested on partial data (11 predictors only) from 29 aircrews. It has a sensitivity of 0.94 and a specificity of 0.75 even when using just 11 predictors that are available before reporting to IAM.
Conclusion
The composite predictive model indicates the relative importance of ongoing evaluations and predicts focus areas affecting aircrew’s return to flying. It also functions as a decision assist tool for the Medical Officer in the field before referral to IAM for evaluation.
Keywords
Bayesian model
Human Engineering
Indian aircrew
Predictive modeling
Spinal disabilities
INTRODUCTION
Spinal disabilities occur in aircrew at the same rate as the normal population, with or without obvious pathology in the spine. Precious man hours are however lost in the diagnosis, treatment, rehabilitation, and assessment for fitness of aircrew each year. Due to the specialized nature of the job, the criteria for assessment and subsequent fitness to fly in the case of aircrew are different from the general population.[1]
Department of Human Engineering, Institute of Aerospace Medicine (IAM) evaluates IAF aircrew with spinal disabilities before they can fly again. Clinical, radiological, and functional evaluations are performed, including the evaluation of aviation-specific simulators. These assessments have proven effective in reflighting aircrew or identifying those who need sheltered duties. This evaluation method has evolved over time. Empirically, it seems to classify aircrew who should return to flying and those who need more management or recovery time.[1-3] Cross-sectional data from the Department of HE show that at any given point in time, while 57% of the aircrew with spinal disabilities may be awarded a flying category, 43% are sent back with a non-flying category.
It is important to standardize this procedure, identify the relative importance of each step, and eliminate redundant evaluation stages. This would improve the ability to predict the outcome of aircrew with a spinal disability and expedite their return to flying, maximizing trained manpower.
This study aimed to develop a Bayesian instrument for spinal disability (BIS) based on clinical, radiological, and functional evaluations of IAM aircrew. The composite model indicates the relative importance of ongoing evaluations and predicts focus areas affecting aircrew’s return to flying. It is also a predictive tool that may be used in the field before referral to IAM.
Aims and objectives
To construct and validate a predictive model for spinal disability assessment in aircrew.
To design a composite model of spinal disability status applicable to aircrew of different streams
To incorporate most factors that determine flying fitness in cases of spinal disability
To validate the predictive value of this scale
To develop a computerized version of the scale that can be used in the field before referral to IAM.
MATERIAL AND METHODS
One hundred twenty aircrews reporting spinal-related disabilities to the Department of Human Engineering, IAM participated in the study. Aircrew with disability in addition to that of the spine was not included in the study as these disabilities are independent predictors for return to flying status.
The aircrew documents available before evaluation at IAM, were perused to tabulate the following data:
Age
Gender
Branch
Stream
Disability
History of low back ache at the onset of disability
Mode of onset of disability
Salient magnetic resonance imaging (MRI) findings on the latest MRI
Modality of treatment (whether surgical or conservative)
Duration of the disease (days since onset of the disease)
Present medical category in which reported to IAM (whether presently in flying or non-flying category).
Following this, the opinion of clinical specialist was sought. Thereafter, their perceived functional status was recorded on short form 36 health survey also known as SF-36 form.[4,5] The SF-36 is an approved measure of the medical outcomes trust, a highly regarded clearinghouse for outcome measures with proven psychometric properties and utility. The SF-36 is subdivided into 2 separate health constructs: The physical component summary score and the mental component summary score. Subscales of the SF-36 measure 8 different health concepts including general health, physical functioning, role functioning, bodily pain, mental health, emotional functioning, vitality, and social functioning.
They underwent functional evaluation as per protocol and opinion of the Aerospace Medicine specialist was then rendered as a binary outcome variable of either flying or ground medical category.
The collected predictor variable data set was used to construct Bayesian Networks (BNs) for classification of the aircrew into an outcome of final medical category of flying or grounded. The BNs were created using GeNie Modeler, developed at the Decision Systems Laboratory, University of Pittsburgh.[6,7]
Note on Bayesian modelling
A Bayesian model is a statistical approach that uses prior beliefs and new data to update the probability of an outcome. It is particularly useful for predictive modeling because it can incorporate diverse factors and does not require very large datasets.
Prior beliefs: Bayesian statistics start with a “prior belief ” about the likelihood of an event. This belief is based on any existing data, knowledge, or even subjective opinion. The prior belief is quantified using a Prior distribution.
New data and likelihood function: When new data are collected, it is used to create a likelihood function, which quantifies how likely the observed data are given a particular hypothesis.
Posterior distribution: The prior distribution is then combined with the likelihood function using Bayes’ theorem to produce a “posterior distribution”. The posterior distribution represents the updated belief about the event given the new data.
Bayes’ theorem: The mathematical basis of Bayesian statistics is Bayes’s theorem which uses conditional probabilities to calculate posterior probabilities. Conditional probability of an event A is the probability of it occurring, given that event B has already occurred.
P (A|B) is the conditional probability of A given B
P (B|A) is the conditional probability of B given A
P (A) and P (B) are the probabilities of A and B occurring independently.
Bayesian models can be used in different ways to predict health outcomes.
BNs. BNs use a graphical approach to depict multivariate probability distributions, showing the probabilistic relationships between multiple variables/events. They are directed acyclic graphs, where nodes represent variables and links represent probabilistic relationships.
Augmented naive bayes (ANB). An ANB network maintains the structure of the Naive BN but adds links between variables to show correlations between the attributes. The ANB is a better descriptor of real-world situations because it is not constrained by the number of dependencies between nodes.
The consistency of the model’s predictions was first validated using the leave one out (LOO) method.[8] Thereafter, the model was independently tested on a data set of 29 aircrews with spinal disabilities for the positive and negative predictive values.
Note on the LOO method
The LOO method is a validation technique used to assess the performance of a predictive model. In the context of this study, it was used to validate the BIS model.
The process. In LOO, the model is trained on n-1 records (where n is the total number of records in the dataset), and then tested on the remaining single record. This process is repeated n times, so that each record in the dataset is used once as a test case.
Model Structure. The model structure which was determined by the BN remains fixed, but conditional probabilities are learned using the n-1 data points in each iteration.
Purpose. This approach helps to evaluate how well the model can generalize to new, unseen data by testing on every data point as a unique test case.
RESULTS
Demographic data
83.3% of the aircrew were below 40 years of age.
Only seven aircrews were female out of the total 120 aircrews in this study.
89.2% of the aircrew were flying pilots. 46.7% of the aircrew in this study were from the helicopter stream.
55% of cases of spinal disability had a documented prolapsed inter-vertebral disc (PIVD). However, more significantly, 40.8% had no clear evidence of radiological abnormality or trauma as the cause for the back pain. 67% of the aircrew in this study had an insidious onset of back pain without being related to a specific traumatic or systemic cause.
The latest MRI of the aircrew showed no nerve root compression in 79.2% of the cases.
DISCUSSION
All the predictive factors were analyzed and the relationships between factors were modeled using the Augmented Naïve Bayes algorithm to decide the final fitness of the aircrew.[9-11] The acyclic graph of the BIS with the current beliefs based on the data of 120 aircrews is presented in Figure 1.

- Acyclic graph showing the current beliefs of all nodes. Note: In this graph, all the variables or “nodes” are depicted with their interrelationships to each other. The inter-relationships or influences are depicted using arrows or “arcs.” The arcs are directional in nature. An arrow leading from a node to another node means that the first node is influenced by the state of the second one. The strength of influence of one node on the other is a function of the conditional probability tables.
Validation of the BIS was carried out using the LOO method. In this, the network was trained on 119 records (n-1) and tested on the remaining one record. The process was repeated 120 times. The model structure was kept fixed, but the conditional probabilities were learned using 119 data points. The resultant network was then tested using the data of the left-out data point. The accuracy was found to be 0.97, sensitivity was 0.97, and specificity was 0.96.
The BIS was also tested on partial data from 29 aircrew. Three important variables/factors, which are generally collected once the aircrew reports to IAM, were excluded from the database. The excluded factors were Clinician’s Opinion, SF 36 Questionnaire score, and the functional evaluation outcome. This data were used to explore the utility of the Bayesian model based on data that are available before the aircrew reports to IAM. The results showed that:
The results show that out of 29 cases, the BN was able to correctly predict the outcome variable state (Flying Category or Ground Medical Category) 25 times. This means that the model has an accuracy ([True Positive + True Negative]/[Total Population]) of 0.86.
It has a sensitivity ([True Positive]/[Actual Positive]) of 0.94 wherein it correctly predicted 16 cases as flying fit out of the 17 cases who had been deemed flying fit as per the data.
It has a specificity of (True Negative/Actual Negative) of 0.75 wherein it correctly predicted 9 cases as ground medical category out of 12 cases who were recommended ground medical category as per the data.
Implications of the BIS
Software version of the BIS. The predictive model/scale has been developed on an open-source platform GeNie Modeler, developed at the Decision Systems Laboratory, University of Pittsburgh, and licensed to BayesFusion, LLC. Academic license for use for academic purposes is available with the authors. Since IAM is the only center for the evaluation of spinal disabilities in the IAF, the BIS is being used at the Department of Human Engineering at IAM.
Individual Scoring and Prediction. The BIS uses demographic characteristics such as gender, age, and stream in its predictive model. It also takes into consideration the specific diagnosis, radiological findings, and treatment. Thus, the BIS scores an individual and gives his probability of upgradation to a flying category based on factors unique to him. This enables follow-up of improvement on an individual basis. It also enables data-based differentiation of final outcomes between two individuals who may be similar in certain aspects of their disability only.
Case study on an individual aircrew. The spinal disability scale applied in one case shows a 95% probability of getting a flying medical category [Figure 2]. Figure 2 shows that in the given case, there is a definite value or state associated with each variable which is a predictor in the spinal disability scale. This particular case is of a 30–40-year-old male aircrew who had reported within the past 1 year with low back ache which was not associated with any trauma or bony lesion. He has no neurological findings on his MRI and has been recommended a flying category by the neurosurgeon. He has scored fairly high on the SF 36 indicating adequate functional status before reporting to IAM. His Human Engineering evaluation is normal. In this case, there is a 95% probability that this aircrew would be considered fit for flying.
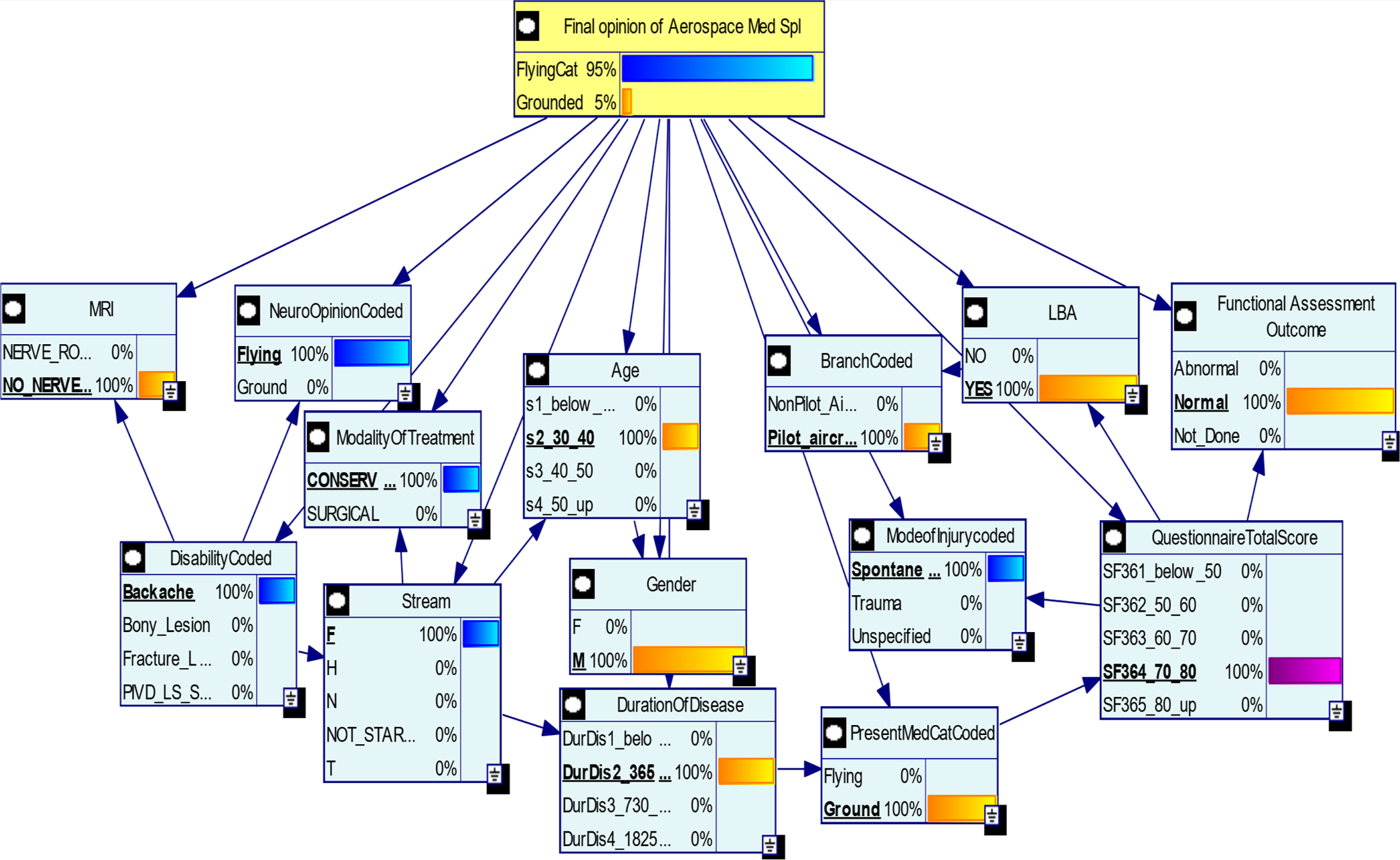
- Outcome prediction of a single case using the Bayesian instrument for spinal-disability model Note: The figure shows that in the given case, there is a definite value or state associated with each variable which is a predictor in the spinal disability scale. In this case, there is a 95% probability that this aircrew would be considered fit for flying.
Recommendations
The following recommendations are made regarding the above study.
The BIS, with its limitations, may be evaluated on a larger number of aircrew with spinal disabilities over a longer period to verify its robustness. It is recommended that the state values of each variable in each new case should be added to the existing database and that the model should be constantly updated.
The BIS with a high negative predictive value with data that are available before the aircrew reports to IAM and hence may be used as a decision-assist tool by Medical Officers in the Field before sending cases for review at IAM.
The current policy in IAF states that all aircrew should be given a permanent medical category within 2 years from the onset of the disability.[12] BIS is likely to be useful for individualized prediction of the probability of flying fitness early in the course of the disease. Based on the prognosis, early decision can be taken regarding the disposition of such cases.
A separate study may be undertaken to include more parameters in the BN so that spinal disability cases with co-morbidities may also be included in the predictive domain of this scale.
CONCLUSION
BIS was constructed using a BN from the data collected of the 120 aircrew who reported to IAM for evaluation. It considered the clinical, functional, and radiological outcomes to predict an aircrew’s fitness during the evaluation at IAM. It takes into consideration various demographic factors including the stream of the aircrew. The spinal disability scale gives individual scores/probabilities of the outcome and can direct targeted follow-up. The BIS showed high accuracy, sensitivity, and specificity during validation. A software-based implementation has been developed and placed in the Department of Human Engineering at IAM.
Ethical approval
The Institutional Review Board has waived the ethical approval for this study.
Declaration of patient consent
The authors certify that they have obtained all appropriate patient consent.
Conflicts of interest
Punyashlok Biswal and Divya Narayanappa is on the editorial board of the journal.
Use of artificial intelligence (AI)-assisted technology for manuscript preparation
The authors confirm that there was no use of artificial intelligence (AI)-assisted technology for assisting in the writing or editing of the manuscript and no images were manipulated using AI.
Financial support and sponsorship: Data from the Department of Human Engineering, Institute of Aerospace Medicine.
References
- A retrospective analysis of musculoskeletal disabilities in military and civil aircrew. Indian J Aerosp Med. 2008;52:8-13.
- [Google Scholar]
- Scoring the SF-36 in orthopaedics: A brief guide. J Bone Jointt Surg Am. 2014;97:1628-34.
- [CrossRef] [PubMed] [Google Scholar]
- Content comparison of questionnaires and scales used in low back pain based on the international classification of functioning, disability and health: A systematic review. Disabil Rehabil. 2012;34:1167-77.
- [CrossRef] [PubMed] [Google Scholar]
- Visualizing inference in Bayesian networks. 2006. Available from: https://www.kbs.twi.tudelft.nl/docs/MSc/2006/JRKoiter/thesis.pdf [Last accessed on 2024 Aug 16]
- [Google Scholar]
- Bayesfusion.com. 2023. Available from: https://support.bayesfusion.com/docs/ [Last accessed on 2024 Aug 16]
- [Google Scholar]
- Performance evaluation of classification algorithms by k-fold and leave-one-out cross validation. Pattern Recognit. 2015;48:2839-46.
- [CrossRef] [Google Scholar]
- Comparing performances of Markov Blanket and tree augmented naïve-bayes on the IRIS dataset In: International multi conference of engineers and computer scientists. 2014. p. :328-31.
- [Google Scholar]
- A new hierarchical redundancy eliminated tree augmented naive bayes classifier for coping with gene ontology-based features. 2016. Available from: https://arxiv.org/abs/1607.01690 [Last accessed on 2024 Aug 16]
- [Google Scholar]
- Applying naive bayesian networks to disease prediction: A systematic review. Acta Inform Med. 2016;24:364-9.
- [CrossRef] [PubMed] [Google Scholar]
- Musculoskeletal disabilities: Chapter 9 of manual of medical examinations and medical boards (6th ed). New Delhi: Indian Air Force; 2020.
- [Google Scholar]