Translate this page into:
Principal component analysis: The path ahead for aircrew-aircraft compatibility at the Institute of Aerospace Medicine
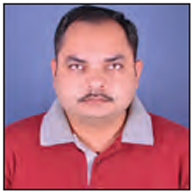
*Corresponding author: Dr P Biswal, (MBBS, MD) Specialist in Aerospace Medicine (Army), Department of Human Engineering, IAM, Bangalore, India. drpunyashlok@gmail.com
-
Received: ,
Accepted: ,
How to cite this article: Biswal P, Dahiya YS. Principal component analysis: The path ahead for aircrew-aircraft compatibility at the Institute of Aerospace Medicine. Indian J Aerosp Med 2019;63(1): 8-15.
Abstract
Introduction:
The Institute of Aerospace Medicine provides design consultancy on aircraft-aircrew compatibility on a number of fixed-wing as well as rotary-wing aircrafts during various stages of development. Till date, the cockpit compatibility of aircrew has been determined based on the percentile concept. Percentiles, though useful when dealing with a single parameter, pose major design and fitment problems when considering multiple parameters simultaneously as in aircraft cockpit design. The concept of multivariate analysis has been the solution which the aviation industry the world over has accepted in overcoming this problem. This paper presents the Institute of Aerospace Medicine initial foray into the field of multivariate analysis, specifically principal component analysis (PCA) to achieve desired aircrew fitment in the aircraft cockpit right from the design stage.
Materials and Methods:
The fighter aircraft of the near future is being designed using the anthropometric parameters available in the IAF aircrew anthropometry survey 2013. Of the 57 parameters available, six parameters critical to the design of the cockpit were subject to PCA to derive three principal components. About 96% confidence ellipse was drawn on the plot of the principal components. From this, along the different axes, 21 boundary individuals were identified defining the extreme individuals in various combinations of the six parameters.
Discussion:
The use of more than 2 parameters is not amenable to sequential use of percentiles. As the number of parameters considered increases, it leads to reduced fitment percentage. The use of PCA allows consideration of critical parameters together at one go. The design aim is changed from the 3rd to 97th percentile to an overall aim of fitting 96% of the target population in the cockpit. The boundary individual’s entire anthropometry data are used to create boundary manikins for use in computer-aided design models. The fitment of these boundary individuals ensures that if these individuals fit, all others would fit in the cockpit. This concept brings about a paradigm shift in the aircrew-aircraft compatibility in the aviation industry in India.
Keywords
Principal component analysis
Anthropometry
Aircrew-aircraft compatibility
Percentile
INTRODUCTION
The Institute of Aerospace Medicine (IAM) provides design consultancy on aircraft-aircrew compatibility for a number of fixed-wing as well as rotary-wing aircrafts during various stages of development. The latest of these is the involvement of the IAM at the “drawing board” stage of the light combat aircraft Mk-2. Till date, cockpit compatibility of aircrew has been determined based on the percentile concept. Percentiles, though useful when dealing with a single parameter, pose major design and fitment problems when considering multiple parameters simultaneously, as in aircraft cockpit design. This has led to difficulty in achieving conflicting design aims and often designing for unoccupied anthropometric zones. The concept of multivariate analysis has been the solution which the aviation industry the world over has accepted in overcoming this problem.[1-5] This paper presents IAMs initial foray into the field of multivariate analysis, specifically principal component analysis (PCA) to achieve desired aircrew fitment in the aircraft cockpit right from the design stage.
The “percentile” problem
The aim of anthropometric fitment is that the user or the designer specifies a certain percentage of the population, which the design of an aircraft cockpit must accommodate. This concept is primarily meant to ensure that the maximum number of aircrew flying a particular aircraft can do so safely and effectively. In this concept, a secondary aim is to avoid designing for those individuals who have extreme anthropometric parameters either large or small so that the most volume economical cockpit fits the maximum number of people.[6]
To achieve this aim, traditionally, a 3rd–97th percentile or 5th–95th percentile fitment is aimed at. Ostensibly, this seems to be a suitable fitment aim, wherein each parameter which is critical for fitment is designed such that the 3rd–97th percentile population of that parameter is able to fly the aircraft safely and effectively. However, in execution of such a concept, the designers and the users have followed a percentile-based univariate model till now.
In univariate percentile concept of fitment, the three critical anthropometric parameters of sitting height, leg length, and thigh length are considered, but sequentially. This means, the 3rd–97th percentile population of each parameter is applied for fitment one by one. Theoretically, this should ensure fitment of approximately 96% of the population, excluding just 4% of the population. Therefore, theoretically, the sequential use of these three parameters would bring the fitment down to 84%. In reality, there exists some overlap of anthropometric parameters depending on their correlation and the actual fitment with three criteria though not necessarily 84% is still well below the expected 96%.
In a study carried out at IAM, the four anthropometric selection parameters of the IAF were applied sequentially to 2445 aircrew aspirants who reported for entry-level medical examination. The results show that selecting 3rd–97th percentile of each of these parameters sequentially reduces the percent of fitment progressively till it reaches 90.8% of the reported population instead of the expected 96% [Figure 1]. As more and more critical parameters are considered, the fitment keeps decreasing progressively, even though, for each anthropometric parameter, the 3rd–97th percentile is being considered.[3]

- Percentage of fitment as number of parameters sequentially considered increases for 2445 aircrew aspirants from 2008 to 2015.
This problem of overall fitment percentage occurs primarily due to a difference in variance between the anthropometric parameters under consideration.
Identifying boundary parameters
Percentile, while defining the boundary parameters of any single parameter, does not take into account the proportional variability with other parameters. This leads to a second problem. The small pilot by the percentile concept is an individual with all parameters at the specified 3rd percentile or a large pilot has all parameters at the 97th percentile. Such individuals do not exist in real life. Trying to design for such individuals introduces fitment regions that demand adjustability for ranges which do not exist in the population. Moreover, there exist certain pilots who would be large on some parameters and short on others, for example, a pilot with a short sitting height but a long leg length would sit high on the seat but with the rudder at the foremost position. This situation is likely to bring his knee closer to the main instrument panel (MIP) than permissible and thus be unsafe, even though he is cleared on each individual parameter.
With two such variables, it is possible to plot both on two different axes and identify an ellipse centered around the common mean which accommodates the desired percentage of the population. A graph of height versus weight of 833 aircrews was plotted [Figure 2]. A fitment ellipse accommodating 96% of the population was drawn. The boundary of the ellipse at its diagonals represents the individuals who are large (point 1) or small (point 2), respectively, in both parameters of height and weight. However, points 3 and 4 are the additional boundary points which identify individuals who are large on one parameter and small on the other. Figure 2 shows that 96% of the 833 aircrews lie within the ellipse. All the individuals who lie at various points on the boundary of the ellipse represent the extremes for different proportional variations for height and weight.

- Bivariate plot of height versus weight showing different body proportion combinations.
This concept may be carried forward to three critical parameters where the third axis would be orthogonal to the first two and the desired fitment percentage would form a three-dimensional (3D) ellipsoid. The number of boundary parameters would now contain additional individuals denoting the different proportions of the three parameters in combination. The addition of a fourth critical parameter leads to the formation of a hyperspace 4D ellipsoid and is not easily plottable. In cases of complex spaces like cockpits, six to 12 parameters may need to be considered when taking into account the canopy clearance, the vision over the nose, the reachability of controls, the width of the cockpit, the distance of knee from MIP, and the ability to operate rudders. While the necessity to consider many parameters simultaneously is established, it is also clear that the statistical methods to establish boundary parameters cannot continue to be the older percentile-based calculations.
Multivariate analysis
The two problems of overall percentage fitment and consideration of proportional variation between parameters can be addressed using a multivariate accommodation method called PCA. PCA is a statistical tool for dimension reduction such that it reduces a large number of variables to two or three more manageable ones. This enables the designers to a select a desired percentage level of the population to be accommodated. This percentage accommodation is done in such a way that not only size variance but also the proportional variability is taken into account.[1,3,7,8]
PCA
PCA is a dimension reduction procedure which reduces the number of parameters needed to describe body size variability by combining a large number of related parameters into a smaller set of factors or components based on their correlation or covariance. From these reduced number of two or three factors, accommodation ellipses or ellipsoids can be drawn for fitting the desired percentage of the population. Once the percentage ellipse is drawn, it is then possible to identify individuals at the boundary of the ellipse who represent not only extreme values in some parameters but also combinations of proportions between parameters that actually exist in the population.[3,4,9]
PCA to identify boundary parameters in IAF fighter aircrew
A study was carried out on the data of 833 fighter aircrews from the IAF anthropometry survey 2013 to identify boundary individuals and their anthropometric parameters. The step-wise methodology of the study, the logic behind each step, and the results derived are presented sequentially in the subsequent paragraphs.
Step 1 (selection of critical or control parameters)
These parameters depend on the design philosophy of the designer and may vary from aircraft to aircraft. However, the basic principles of reaches and clearances remain universal. This study empirically uses six anthropometric parameters which are considered critical for the fitment of an aircrew in a fighter aircraft cockpit. These parameters are listed in Table 1.
Anthropometric parameters | Cockpit criticality |
---|---|
Sitting height | Overhead clearance |
Eye-level height | Internal and external vision |
Acromion shoulder height | Starting point for all reaches |
Arm reach thumb tip /knuckle | Reach to controls |
Thigh length | Ejection path clearance and reach to rudders |
Knee height | Ejection path clearance and reach to rudders |
Step 2 (standardization of data)
PCA is an exercise in maximizing variance among the control parameters. Raw data may have different variance due to the difference in the scales of data. For example, a parameter such as sitting height may show a larger variance than a smaller parameter such as thigh length, leading to bias toward the larger parameter in the PCA. Standardization of data is done by calculation of “Z” scores for that parameter which is then used for PCA.[3]
Step 3 (PCA)
The PCA analysis was carried out using JASP v0.10.2 and PAST v3.2. The principal component factor loadings are given in Table 2. The same is represented graphically in Figure 3a-c.
Anthropometric parameters | PC 1 | PC 2 | PC 3 | PC 4 | PC 5 | PC 6 |
---|---|---|---|---|---|---|
Sitting height | 0.46 | −0.33 | 0.09 | −0.04 | −0.23 | −0.78 |
Eye-level height | 0.44 | −0.38 | 0.03 | 0.21 | −0.53 | 0.57 |
Acromion shoulder height | 0.43 | −0.37 | 0.04 | −0.16 | 0.78 | 0.20 |
Arm reach knuckle | 0.38 | 0.35 | −0.83 | 0.17 | 0.05 | −0.04 |
Thigh length | 0.35 | 0.51 | 0.50 | 0.58 | 0.15 | −0.02 |
Knee height | 0.37 | 0.47 | 0.19 | −0.74 | −0.18 | 0.13 |

- (a) Factor loading principal component 1. (b) Factor loading principal component 2. (c) Factor loading principal component 3.
The factor loadings in Table 2 represent covariance of variables with the principal components. The principal components show the following factors/descriptors of the entire population Table 3.
Components | Eigenvalues | ||
---|---|---|---|
Total | % of variance | Cumulative % | |
1 | 3.560 | 59.333 | 59.333 |
2 | 1.250 | 20.834 | 80.167 |
3 | 0.448 | 7.467 | 87.634 |
4 | 0.374 | 6.238 | 93.872 |
5 | 0.250 | 4.163 | 98.034 |
6 | 0.118 | 1.966 | 100.000 |
The loadings on the first component or factor are all positive and uniformly loaded across all variables/ parameters. PC1 describes the overall size of the individuals
The positive and negative loadings of the second factor PC2 contrast people with long limb/short torso body proportions and people with short limb/long torso body proportions
PC3 shows the contrast/variation in people with long arms/short thighs and people with short arm/long thighs.
Together these three principal components explain 87.63% of the variation existing in the population due to the six parameters. Adding more components would add to just 12% of the explained variance while adding complexity to the model and is therefore ignored. A scree plot of the principal components and variance explained is shown in Figure 4 which shows an “elbow” at three components.[3,9]

- Scree plot of principal components and variance explained.
Step 4 (plotting of data points and confidence ellipse/ ellipsoid)
Once the number of principal components is defined, this identifies the principal component space within which the anthropometric fitment can be carried out. With three identified principal components in this study, three orthogonal axes of PC1, PC2, and PC3 were plotted to define a three-dimensional space. Data from the population (833 fighter pilots) under consideration were plotted in this 3D space [Figure 5]. In this study, the non-fitment of 2% very small and 2% very large was considered acceptable (as per philosophy of fitting 3rd–97th percentile). A 96% confidence ellipsoid was plotted in the 3D space such that it encompassed 96% of the population under consideration [Figure 6]. The spheroid was drawn using the Cartesian coordinate equation of a triaxial ellipsoid centered at the origin with semi-axes a, b, and c aligned along the coordinate axes: . The values of a, b, and c are derived from 96% of the range of the principal components PC1, PC2, and PC3, respectively.[10] The data points that appear in blue color outside the green spheroid in Figure 6 are the ones which had parameters outside the 96% fitment model and hence did not fit and were excluded.

- Data plotted along the three principal components.

- 96% Accommodation boundary confidence ellipsoid plotted.
Step 5 (identification of boundary case individuals)
The 96% confidence spheroid/ellipsoid is useful for visualizing the rejected data points. However, it can be appreciated that there exist some empty spaces in certain regions of the spheroid. The boundary individuals or data points do not coincide with the surface of the sphere in the cardinal directions. Therefore, in this study, three biplots plotting PC1 versus PC2, PC2 versus PC3, and PC1 versus PC3 were used to draw boundary ellipses and identify the boundary individuals.[3] This technique uses the same concept of confidence ellipse in the two-dimensional (2D) plane. It also utilizes a statistical technique called “minimum spanning tree” which minimizes the length of the edges of the graph. This helps in identifying those data points which are closest to the surface of the 96% confidence spheroid.[11] The three plots yielded eight boundary individuals each, at intersection of principal components, leading to 24 boundary individuals/data points in Figure 7a-c. These 24 points being derived from 2D plots were cross-validated with the 3D ellipsoid. Data point no. 13 which appeared to be a boundary point in PC1 versus PC3 was rejected as it actually lays well outside the spheroid. Data points 16 and 17; 1 and 19 are of the same individuals. Therefore, one each of these was also deleted from the final list. This finally leads to 21 data points which describe the boundary individuals in all possible dimensions and proportions for the basic six parameters used in the cockpit design. The data are presented in Figure 8.

- (a) Boundary points 1–8 from PC1 versus PC2. (b) Boundary points 9–16 from PC1 versus PC3. (c) Boundary points 17–24 from PC2 versus PC3.

- Boundary Individuals with measurements of critical parameters in cms, graph of standardised measurements and description of body proportions from the Fighter Aircrew population of IAF aircrew Anthropometry survey 2013.
Application of PCA to cockpit design
The boundary individuals identified from the entire available anthropometry data can be used to create boundary manikins for use in computer-aided design (CAD) models. These individuals provide boundary guidelines for designers to determine the positions of critical design parameters such as location of the DEP, clearance from the canopy, location of controls in Zone 1, location of the MIP, and the location/ adjustability of rudders. It is essential for the designers to cater for tolerance zones for ejection clearance as per Mil 1333B when considering individual anthropometric dimensions in design.
Fitment of the boundary individuals ensures that if these individuals fit, all others would fit in the cockpit. Therefore, in the CAD models used in 3D designing, 3D scanned images of only these boundary individuals can be used to create 3D manikins which can be used for fitment. Later, in the cockpit mock-up and prototypes, only these individuals need to be given actual confirmatory trials. It is important to note that individuals who fall outside the confidence ellipsoid are excluded on the basis of simultaneous consideration of all their parameters along with proportions. The excluded individuals may individually be compatible on a number of or even all of these critical anthropometric parameters.
This study shows that the selection of critical parameters is the most important step in applying multivariate analysis to a design problem. The parameters selected must characterize the cockpit environment and the combination of parameters must be relevant to developing adjustability range within the space.
It is also important to decide on the threshold for acceptable level of explained variance. This determines the level of complexity of the model vis-a-vis encompassing maximum variation in the population. In this study, the selection of the first two principal components would provide explained variance of overall body size and proportion of trunk versus limbs. This would also yield only eight boundary individuals. This model would have been simple and the boundary ellipse could be drawn in 2D without involving complex 3D geometry. However, it is only with the addition of the third component that the relative proportions between the arm reach and thigh length could be included in the model. In a cockpit, the arm reach is important for reach of controls while the thigh length is critical for ejection path clearance.
Therefore, at the cost of a more complex model with more than 20 boundary individuals, the three-axis model was considered more representative of the target population.
CONCLUSION
Univariate design poses little problem for the designer when designing simple workspaces/equipment with one or two critical parameters. However, the use of more than 2 parameters is not amenable to sequential use of percentiles. As the number of parameters considered increases, it leads to reduced fitment percentage. The use of PCA allows consideration of all critical parameters together at one go. The design aim, therefore, is changed from an unachievable “3rd–97th percentile in all parameters” to an overall aim of “fitting 96% of the target population” in the cockpit.
The concept of multivariate analysis using PCA brings about a paradigm shift in aircrew-aircraft compatibility in the aviation industry in India.
Financial support and sponsorship
Nil.
Conflicts of interest
There are no conflicts of interest.
References
- Multidimensional Consideration of Anthropometric Diversity. Lyon, France: Proceedings of the 1st International Symposium on Digital Human Modeling; 2011.
- Digital Human Modeling for Indian Anthropometry. Tokyo, Japan: Asian Workshop on 3D Body Scanning Technologies; 2012.
- [CrossRef] [PubMed]
- The USAF multivariate accommodation method. Proc Hum Factors Ergon Soc Annu Meet. 1998;42:722-6.
- [CrossRef] [Google Scholar]
- Cockpit Flight Deck Design : What is the Best Method for Defining Anthropometric Design Criteria? p. 1-8. Available from: https://www.linkedin.com/pulse/cockpit-flight-deck-design-what-best-method-defining-gilvan [Last accessed on 2018 Jul 21]
- [Google Scholar]
- Derivation of boundary manikins: A principal component analysis. Available from: http://www.papers.sae.org/2008-01-1879 [Last accessed on 2018 Jul 21]
- [CrossRef] [Google Scholar]
- Statistical considerations. In: Anthropometric Source Book. (Anthropometry for Designers). Vol 1. Washington DC (USA): NASA Reference Publication; 1978. p. :1024.
- [Google Scholar]
- Description of boundary case methodology for anthropometric diversity consideration. Int J Hum Factors Model Simul. 2012;3:204-23.
- [CrossRef] [Google Scholar]
- A Multivariate Anthropometric Method for Crew Station Design. Report No AL- TR-1993-0054. Armstrong Laboratory. Air Force Material Command, Wright-Patterson Air Force Base, Kent(Ohio); Published by
- [Google Scholar]
- Generation of Boundary Manikin Anthropometry. Available from: http://www.sae.org/technical/papers/2008-01-2107 [Last accessed on 2008 Jul 23]
- [CrossRef] [Google Scholar]
- Handbook of Mathematics and Computational Science. (3rd ed). New York: Springer; 2006. p. :111-7.
- [Google Scholar]
- Past: Paleontological statistics software package for education and data analysis. Palaeontol electronica. Available from: https://www.palaeo-electronica.org/2001_1/past/issue1_01.htm [Last accessed on 2018 Jul 30]
- [Google Scholar]